Understanding Machine Learning: A Deep Dive into Its Business Potential
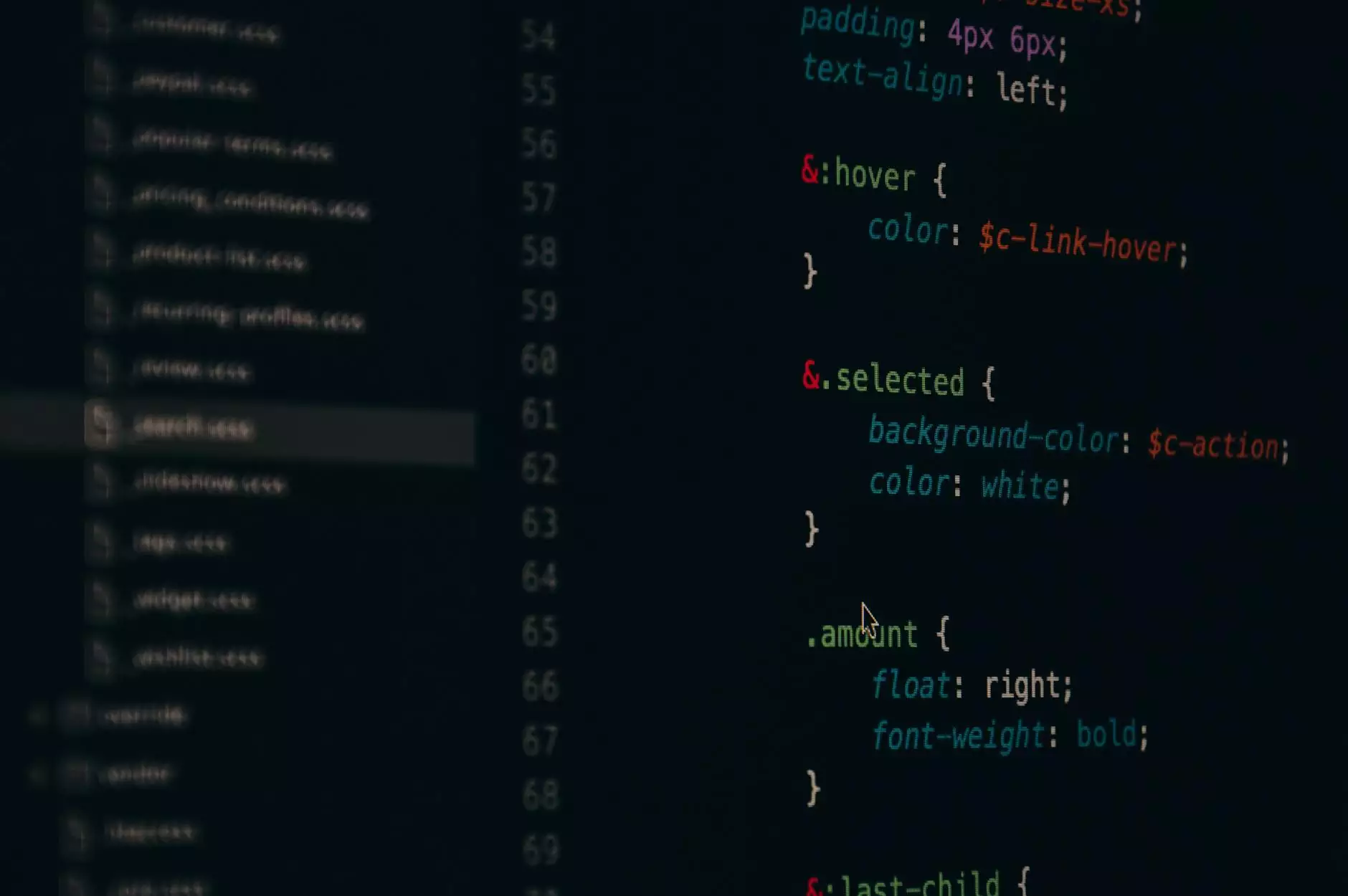
Machine Learning, a subset of artificial intelligence, is revolutionizing the way businesses operate and make decisions. In today's fast-paced digital landscape, understanding information about machine learning is crucial for organizations aiming to maintain a competitive edge. This article will explore various facets of machine learning, its applications, challenges, and future trends in the business world.
The Fundamentals of Machine Learning
At its core, machine learning involves the development of algorithms that allow computers to learn from and make predictions based on data. By leveraging statistical techniques, machines can improve their performance on a given task without being explicitly programmed. This section provides a foundational understanding of machine learning, its types, and how it is employed in business.
Types of Machine Learning
Machine learning can primarily be classified into three categories:
- Supervised Learning: This type involves training a model on a labeled dataset, enabling the machine to predict outcomes for new, unseen data. It is widely used in applications such as fraud detection and stock price prediction.
- Unsupervised Learning: Here, the model processes unlabeled data to discover hidden patterns or intrinsic structures. Common uses include customer segmentation and market basket analysis.
- Reinforcement Learning: This iterative learning process involves agents that take actions in an environment to maximize some notion of cumulative reward. It is notably applied in robotics and gaming.
Key Components of Machine Learning
To fully grasp the efficacy of machine learning in business, it is essential to understand its key components:
- Data: The foundation of machine learning is data. Organizations generate vast amounts of data every day, and harnessing this data is pivotal.
- Algorithms: Algorithms are the mathematical procedures that process data to learn and make forecasts. Choosing the right algorithm significantly influences the output.
- Computational Power: Advanced computational power, provided by GPUs and cloud technologies, enables extensive data processing and complex model training.
- Domain Expertise: Understanding the specific business domain helps in making informed decisions about feature selection and interpretation of results.
Applications of Machine Learning in Business
The application of machine learning spans across various industries, transforming operations and enabling data-driven decision-making. Below are key areas where businesses can benefit from machine learning:
1. Customer Relationship Management (CRM)
Machine learning enhances CRM by analyzing customer data to predict behaviors and preferences. This leads to personalized marketing strategies, enhanced customer service, and improved customer retention rates.
2. Financial Services
In the financial sector, machine learning algorithms are utilized for credit scoring, risk assessment, and fraud detection. By examining transaction patterns, businesses can quickly identify potentially fraudulent activities.
3. Human Resources
Machine learning assists in optimizing hiring processes through tools that analyze resumes and predict candidate success. Additionally, it can assess employee performance and identify training needs.
4. Supply Chain Management
Predictive analytics powered by machine learning improves supply chain efficiency by forecasting demand, optimizing inventory levels, and anticipating potential disruptions.
5. Healthcare
In healthcare, machine learning is employed for predictive analytics, diagnostics, and personalized medicine. It aids in early disease detection and the development of tailored treatment plans.
Challenges of Implementing Machine Learning
Despite its numerous benefits, implementing machine learning in business comes with challenges that organizations must navigate. Understanding these challenges is vital for successful integration:
Data Quality and Quantity
High-quality, diverse, and sufficient data are prerequisites for training effective machine learning models. Poor data leads to inaccurate predictions and can misguide business decisions.
Understanding Algorithms
The selection of appropriate algorithms requires thorough understanding and expertise. Incorrect algorithm choice can result in subpar model performance, highlighting the importance of domain knowledge.
Ethical Considerations
There are ethical implications related to bias in machine learning models. If training data reflects societal biases, the model may perpetuate or exacerbate these biases in decision-making processes.
Integration with Existing Systems
Integrating machine learning solutions with existing business systems can be complex. Organizations must ensure interoperability and scalability to maximize returns on their investment.
The Future of Machine Learning in Business
As technology evolves, the future of machine learning looks promising. Emerging trends that are shaping the landscape include:
1. Enhanced Automation
With advancements in machine learning, businesses can automate repetitive tasks, allowing employees to focus on higher-value activities.
2. Real-time Analytics
The growth of real-time data processing will empower businesses to make timely decisions based on current data rather than relying on historical insights.
3. Natural Language Processing (NLP)
NLP technologies are enhancing customer interaction through chatbots and virtual assistants, improving customer service and engagement.
4. Continued Investment
Organizations will increasingly invest in machine learning capabilities, recognizing its potential for driving innovation and efficiency.
How to Get Started with Machine Learning in Your Business
For businesses looking to adopt machine learning, here are steps to consider:
- Identify Use Cases: Begin by identifying specific business problems that can be solved with machine learning.
- Assess Data Availability: Evaluate the quality and quantity of available data for the identified use cases.
- Build a Skilled Team: Assemble a team of data scientists, analysts, and engineers who can develop and implement machine learning solutions.
- Start Small: Pilot small projects to test the effectiveness of machine learning solutions before scaling up.
- Focus on Continuous Learning: Machine learning is an evolving field; invest in continuous education and training for your team.
Conclusion
As we have explored, the information about machine learning reveals not only its transformative potential across various sectors but also its capabilities to drive efficiency, innovation, and strategic decision-making in businesses. By understanding the fundamentals, overcoming challenges, and harnessing the latest advancements, organizations can position themselves at the forefront of this technological revolution. The journey into machine learning is not only a technical one but also a strategic imperative that can redefine the future of business.